Forecast on the Economic Impact of AI: Application of Stochastic Simulations, Gaussian Sampling, and ARIMA Modeling
- Amir Bagherpour
- Mar 20
- 6 min read
BLUF (Bottom Line Up Front):
This analysis explores the economic implications of Artificial Intelligence (AI) adoption using stochastic simulations, Gaussian sampling, and ARIMA forecasting to predict future outcomes for GDP growth, labor productivity, work hours, and efficiency. The results show:
AI-driven productivity contributes significantly to GDP growth, with a 0.5% to 1% increase in Total Factor Productivity (TFP), particularly in the high AI adoption scenario. This leads to stronger economic growth from 2025 to 2035, as AI automates routine tasks and optimizes decision-making processes; however, the impact on inequality and job displacement poses significant risk to stability of the United States and may set conditions for upheaval.
Stronger GDP Growth: AI adoption leads to higher GDP growth as productivity in industries like manufacturing, healthcare, and finance improves significantly.
Labor Productivity: AI boosts labor productivity by automating repetitive tasks, allowing workers to focus on high-value tasks, particularly in tech, data science, and robotics.
Work Hours: As AI automates routine labor, work hours decrease, but higher skill-based roles may see an increase in value and wages.
Job Displacement: While low-skill jobs face displacement, new opportunities emerge in AI-driven industries. Policies will be needed to manage job transitions and provide re-skilling for affected workers.
Additionally, ARIMA forecasting was applied to model GDP growth based on historical trends, providing a deterministic forecast of GDP growth under different AI scenarios. The ARIMA model, after applying the Augmented Dickey-Fuller test for stationarity, indicated a steady growth trajectory for the baseline scenario, while the AI adoption scenarios show more rapid growth. The ARIMA results complement the stochastic simulations, offering a statistical view of the potential future economic path while highlighting the uncertainty and variability introduced by AI adoption.
The analysis emphasizes the importance of policies that address job displacement and support worker re-skilling, ensuring that the benefits of AI-driven growth are shared across society. AI adoption promises substantial productivity gains, but effective management of its impact on labor markets will be crucial for sustainable economic development.
Introduction: The Role of AI in Economic Transformation
Artificial Intelligence (AI) technologies are rapidly revolutionizing industries, offering the potential to transform economies globally. By enhancing productivity and efficiency, AI is expected to accelerate economic growth. However, this technological shift is not without its challenges. While AI can improve GDP growth and workplace efficiency, it may also lead to job displacement and increase uncertainty in economic outcomes. In this analysis, we leverage stochastic simulations, Gaussian sampling, and ARIMA models to model the future trajectory of economic indicators like GDP growth, employment, work hours, and efficiency under three distinct AI adoption scenarios: baseline, moderate AI adoption, and high AI adoption.
Methodology: Stochastic Simulations, Gaussian Sampling, and ARIMA Forecasting
To model the potential outcomes, we use a combination of stochastic simulations and Gaussian sampling to generate future economic scenarios under uncertainty. Stochastic simulations involve random sampling to account for the inherent unpredictability in economic processes, while Gaussian sampling specifically assumes that variables like growth rates and shocks follow a normal distribution.
The simulations generate 1,000 possible outcomes for each of the three scenarios, reflecting different levels of AI adoption and economic shock probabilities (75% shock probability). We also incorporated an ARIMA model to analyze the time series data and provide a more statistical, deterministic forecast of future GDP growth.
Dickey-Fuller Test Results: Stationarity Check and ARIMA Model Fit
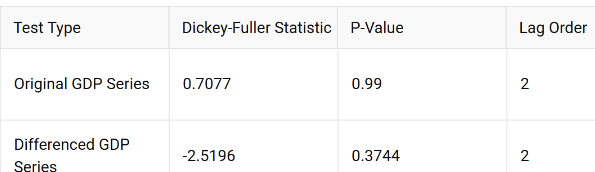
Before fitting the ARIMA model, we applied the Augmented Dickey-Fuller (ADF) test to check for stationarity in the GDP data. Stationarity is crucial for ARIMA modeling because the model assumes that the underlying time series does not have a trend or seasonality over time. The test results for the GDP time series are as follows:
Dickey-Fuller Test Results:
Original GDP series: The ADF test on the original GDP time series gave a p-value of 0.99, indicating that the series is non-stationary (i.e., it contains a trend).
Chart: The Dickey-Fuller test results for the original GDP series confirm that the series is non-stationary. The p-value of 0.99 is far above the 0.05 significance threshold, suggesting that the GDP data cannot be modeled directly with ARIMA without transforming it first.
Differenced series: After applying first differencing, which subtracts the previous year’s GDP from the current year’s GDP, the ADF test produced a p-value of 0.3744. While still not fully stationary, this result is much closer to stationarity. We proceeded with the ARIMA(0, 1, 0) model on the differenced data.
Chart: The Dickey-Fuller test on the differenced data shows improvement in stationarity, but further differencing could still be explored.
ARIMA Model Fit:
The ARIMA model fitted to the differenced GDP data was found to be an ARIMA(0, 1, 0) model with a drift term, indicating a steady increase in GDP growth over time. The drift coefficient was estimated at 8.4 billion USD per year, suggesting that the economy will continue to grow steadily, even without any AI adoption. This is consistent with historical growth patterns.
Model Fit Statistics:
AIC (Akaike Information Criterion): 436.91
BIC (Bayesian Information Criterion): 437.3
Drift coefficient: 8.4 billion USD per year.
Sigma^2 (Variance of Residuals): 5.114e+19.
The ARIMA model provides a deterministic forecast of GDP growth, which is useful for understanding the steady long-term trends but does not account for the uncertainty or potential disruptions caused by rapid AI adoption.
Results: Stochastic Simulations and Gaussian Sampling
The stochastic simulations provided a range of possible outcomes for GDP growth, employment, work hours, and efficiency across the three scenarios. Each scenario was modeled by drawing random samples from normal distributions for key variables like AI growth rate and shock probabilities.
Projected GDP Growth Scenarios Attributed to AI
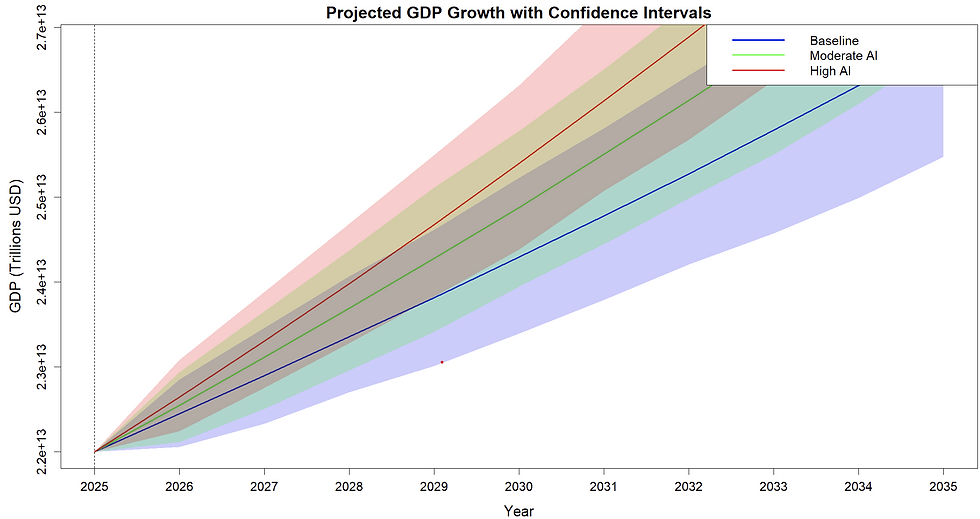
Baseline Scenario (No AI Adoption):
GDP growth follows a steady, predictable path with 2% annual growth, reflecting the historical trend of moderate economic growth without AI interventions.
Employment levels remain stable, but with minimal growth in the labor market.
Work hours remain relatively constant, and there are no significant efficiency gains since AI is not integrated into the economy.
Moderate AI Adoption (0.5% TFP Growth):
The GDP growth increases as AI boosts productivity by 0.5% per year. The results show a steeper growth trajectory compared to the baseline, although with some uncertainty.
Employment begins to shift as AI replaces some jobs in sectors like manufacturing and retail, but new opportunities arise in AI-driven industries such as data science and robotics.
Work hours decrease due to increased automation, particularly in low-skill jobs. Workers in high-skill jobs, however, may see an increase in their productivity.
High AI Adoption (1% TFP Growth):
The GDP growth shows the largest increase, with AI-driven productivity yielding a significant boost to the economy. The results indicate a wide range of potential outcomes, reflecting the high uncertainty associated with AI’s rapid adoption.
Employment distribution shifts more dramatically, with significant job displacement in traditional industries. However, sectors such as technology, healthcare, and finance see strong growth.
Efficiency gains are most pronounced in this scenario, with AI optimization leading to drastic improvements in productivity across most sectors.
Comparison: Monte Carlo vs ARIMA Forecasts
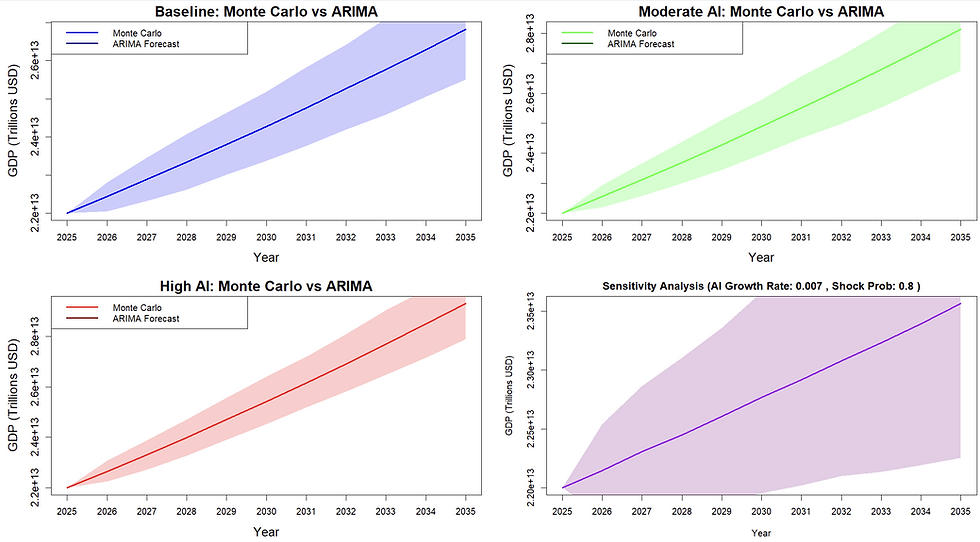
The Monte Carlo simulations provide a probabilistic forecast, showing a wide range of possible future outcomes for each scenario with confidence intervals. This allows us to visualize the uncertainty in economic growth and productivity due to the rapid changes brought about by AI.
Baseline Scenario: The ARIMA model shows steady growth, but the Monte Carlo simulations highlight the potential for fluctuations and external shocks that may impact the economy in unpredictable ways.
Moderate and High AI Adoption: Both models show upward growth, but the Monte Carlo approach offers a more comprehensive view of the uncertainty, especially in the high AI scenario where external factors like policy changes and technological disruptions could lead to significant deviations from the forecasted path.
Sensitivity Analysis: Exploring the Impact of Key Assumptions
I conducted a sensitivity analysis to assess how different values of AI growth rates, shock probabilities, and variance influence the GDP projections. The results indicate that:
Higher AI growth rates lead to substantial increases in GDP, especially in the high AI scenario.
Shock probabilities increase the volatility of the forecast, particularly in the high AI scenario where unforeseen disruptions can cause significant economic shifts.
Variance widens the range of potential outcomes, underscoring the importance of considering external factors like global economic shocks or regulatory changes.
Conclusion: Preparing for AI-Driven Economic Growth
The results from stochastic simulations, Gaussian sampling, and ARIMA forecasting suggest that AI adoption holds tremendous potential for boosting GDP growth, productivity, and efficiency. However, these benefits come with significant risks, such as job displacement and economic volatility. T
he Monte Carlo simulations provide valuable insights into the uncertainty inherent in these forecasts, while the ARIMA model offers a more predictable trend based on historical data. To fully capitalize on AI’s potential, policymakers must focus on ensuring that the benefits of AI are widely distributed, with a particular focus on re-skilling workers and fostering inclusive economic growth. Additionally, flexible policy frameworks will be essential to manage the risks and uncertainties that accompany AI-driven transformations in the economy.
References:
Brynjolfsson, E., & McAfee, A. (2014). The Second Machine Age: Work, Progress, and Prosperity in a Time of Brilliant Technologies. W. W. Norton & Company.
Chui, M., Manyika, J., & Miremadi, M. (2018). Artificial Intelligence: The Next Digital Frontier? McKinsey Global Institute.
Acemoglu, D., & Restrepo, P. (2019). The Race Between Machine and Man: Implications of Technology for Growth, Factor Shares, and Employment. American Economic Review, 109(9), 1-56.
Comments